AI and Predictive Analytics: Shaping Decisions Across Sectors
Exploring the balance between generative AI and predictive analytics, this write up highlights the transformative potential, ethical considerations, and practical applications in shaping data-driven decisions across the public sector.
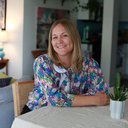
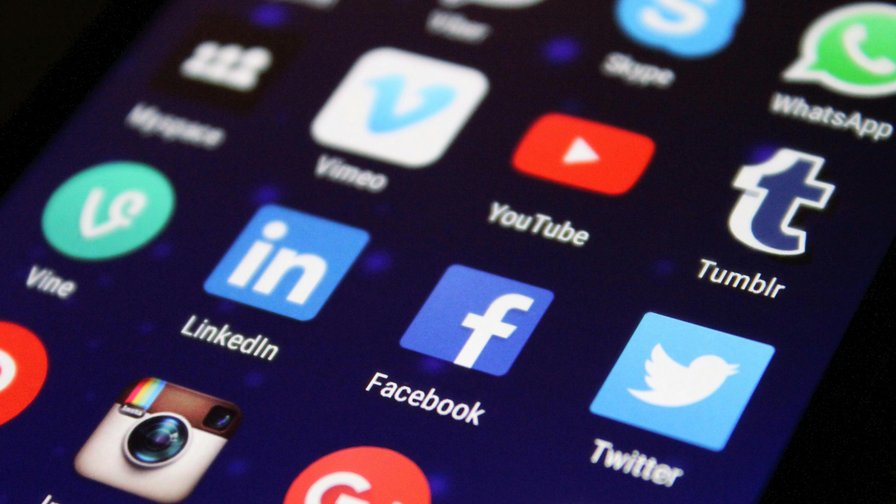
This write up is based on panel session titled AI and Predictive Analytics: Shaping Decisions Across Sectors. It brought together experts from various fields to discuss the evolving landscape of artificial intelligence (AI), predictive analytics, and generative AI in the public sector. The session was facilitated by Ivan Novick, Portfolio Product Manager at VMware by Broadcom, and featured insights from Yvan Gauthier (National Research Council), Meagan Collins (Treasury Board of Canada Secretariat), Cedric Jean-Marie (Canadian Heritage), and Brett Tackaberry (Google Cloud Public Sector). The discussion revolved around determining when to use predictive analytics versus generative AI, enhancing decision-making through data-driven insights, and addressing public sector challenges proactively.
When to Use Predictive Analytics vs. Generative AI
When should organizations choose predictive analytics over generative AI, and is AI the solution for everything?
"Generative AI is not the solution to everything. It’s just a small subset of AI techniques. For structured data or tasks requiring explainability, traditional methods are often better." Yvan Gauthier
Yvan emphasized that generative AI, while powerful, is not always the right tool. For tasks like predictive analytics or recommendation engines, traditional statistical methods are more cost-effective and reliable. He also highlighted the environmental and financial costs of using large language models (LLMs) for simple problems, noting that simpler techniques often provide equally effective solutions.
Cedric added that the choice of tool depends on the nature of the task. For administrative decisions requiring explainability, traditional algorithms are preferable. However, for creative tasks like content generation, generative AI can be highly effective.
"If you’re in the administrative decision space, explainability is key. But for creative tasks, generative AI can be a game-changer." Cedric Jean-Marie
Meagan echoed this sentiment, stressing the importance of educating clients about the appropriate use of AI tools. She compared the decision-making process to choosing the right tool from a toolbox, emphasizing that not every problem requires a high-cost AI solution. She also highlighted the need for cost-effectiveness, as clients often don’t fully understand the financial implications of using advanced AI tools.
Real-World Use Cases: Generative AI and Predictive Analytics
The panelists shared several examples of successful applications of generative AI and predictive analytics in government operations, showcasing the transformative potential of these technologies.
Generative AI in Action
Canadian Heritage: Cedric discussed an experiment using generative AI to streamline ministerial correspondence. The AI-generated responses were faster, more grammatically accurate, and often indistinguishable from human-written letters. The experiment demonstrated significant time savings and improved quality, with the AI producing responses in half the time of human authors.
National Research Council: Yvan highlighted the use of an internal chatbot, AI Zone, which helps staff summarize information about Canadian businesses, improving efficiency in meetings and decision-making. The chatbot leverages generative AI to pull relevant data, such as patents and previous agreements, enabling staff to prepare more effectively for meetings.
Predictive Analytics Success Stories
Skate Canada: Cedric shared an example where predictive analytics was used to allocate micro-grants efficiently. The algorithm ensured that administrative costs did not exceed the funds being distributed, optimizing resource allocation and ensuring that more funds reached the community.
Coding and Project Management: Meagan mentioned how generative AI tools like Copilot are helping coders and project managers save time by generating code frameworks and project charters, enabling teams to do more with less. She also noted that these tools are particularly useful for tasks like drafting emails and improving language skills, especially in bilingual environments.
Risks and Ethical Considerations
The panel also delved into the risks associated with AI and predictive analytics, particularly around data integrity, bias, and explainability. The discussion highlighted the importance of ethical stewardship and the need for robust governance frameworks.
"We need to be stewards of the data we collect. Historical data can carry systemic biases, and we must be mindful of the ethical implications." Meagan Collins
Meagan emphasized the importance of multidisciplinary teams to address ethical concerns, ensuring that data usage aligns with legal and privacy standards. She also called for updated consent structures to reflect the evolving use of data in AI applications, noting that traditional consent forms may not account for the use of data in AI models.
Yvan added that clear guidelines and tools are essential to mitigate risks. He stressed the need for secure environments where employees can experiment with AI without resorting to unauthorized tools like ChatGPT.
"Humans are flawed, and so is the data we generate. We need to acknowledge these flaws and build processes to detect and respond to them." Cedric Jean-Marie
Brett Tackaberry highlighted the importance of integrating security controls into AI systems, ensuring that data used for training models is continually monitored and protected. He also emphasized the need for transparency in how AI systems make decisions, particularly in high-stakes areas like public safety and economics.
Explainability and Transparency
A key concern raised during the session was the explainability of AI-driven decisions, particularly in high-stakes areas like public safety and economics. The panelists agreed that explainability is not just a technical requirement but also a moral and legal obligation.
"You are required to explain your decisions, whether they’re made by algorithms or humans. People must also have the ability to challenge those decisions." Cedric Jean-Marie
Yvan noted that while advanced techniques like mechanistic interpretability are being explored, simpler, explainable methods are often more appropriate for administrative decisions. He cautioned against using "black box" models in situations where transparency is critical.
Meagan called for greater transparency in how data is used, advocating for open-source code and clear communication with the public about AI applications. She also highlighted the need for ongoing dialogue with stakeholders, including Indigenous communities, to rebuild trust and ensure that data is used responsibly.
Audience Questions: Training and Protected Information
The session concluded with questions from the audience, focusing on training employees to use AI tools effectively and detecting protected information. The panelists provided practical insights into how organizations can address these challenges.
Training for AI Tools: Yvan acknowledged the need for better training programs, including prompt engineering and digital literacy. He noted that while there are many free resources available, organizations should also invest in tailored training programs to meet their specific needs. Cedric shared insights from Canadian Heritage’s experiment, where regular feedback sessions helped employees improve their use of generative AI over time. He emphasized the importance of creating a culture of experimentation and learning, where employees feel comfortable exploring new tools and techniques.
Detecting Protected Information: Brett explained how cloud providers use AI to detect sensitive information by identifying patterns. This capability can be used to automatically tag or redact protected data, enhancing security and compliance. He also highlighted the potential for generative AI to assist with tasks like redacting sensitive information from documents, reducing the burden on human reviewers.
Key Takeaways
Choose the Right Tool: Generative AI is powerful but not always the best solution. Predictive analytics and traditional methods remain essential for structured data and explainable outcomes.
Ethical Stewardship: Organizations must address biases in historical data and ensure transparency in AI-driven decisions. This requires multidisciplinary collaboration and ongoing dialogue with stakeholders.
Invest in Training: As AI tools become more prevalent, training employees to use them effectively is crucial. Organizations should provide both technical training and guidance on ethical considerations.
Collaborate Across Departments: Leveraging expertise from across government can accelerate AI adoption and mitigate risks. Shared services and horizontal collaboration are key to success.
Explainability Matters: Decisions made by algorithms must be explainable, especially in high-stakes areas. Organizations should prioritize transparency and accountability in their AI strategies.
Guardrails and Governance: Establishing clear guidelines and guardrails is essential to ensure responsible AI use. This includes creating secure environments for experimentation and integrating security controls into AI systems.
The panel session underscored the transformative potential of AI and predictive analytics while highlighting the importance of ethical considerations, transparency, and collaboration. As governments continue to adopt these technologies, the insights shared by the panelists will be invaluable in shaping responsible and effective AI strategies. By choosing the right tools, investing in training, and prioritizing ethical stewardship, organizations can harness the power of AI to drive innovation and improve service delivery for citizens.
- Related Events
- Government Innovation Week Federal
- Communities
- General
- Regions
-
Canada
Published by
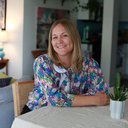
Related
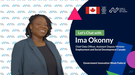
Interviews
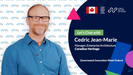
Interviews
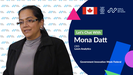
Interviews
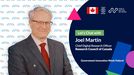
Interviews
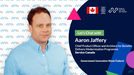
Interviews
Recommended
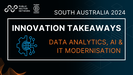
Industry Trends
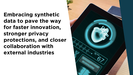
Case Studies
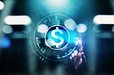
Industry Trends
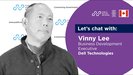
Interviews

Industry Trends
Sign up
Most Popular Insights
Most Popular Partner Content