Case Study: Supporting Frontline Clinicians in SA through Data-Driven Decision Making
A new learning health system to automate decision-making processes and reduce the cognitive load on clinicians for South Australia
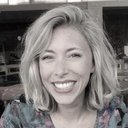
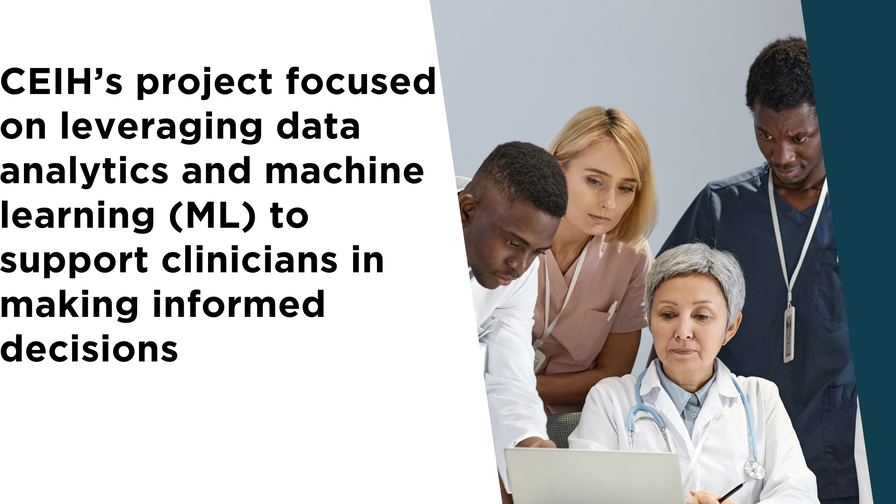
With Tina Hardin, Executive Director, Clinical Informatics and Innovation, the Commission on Excellence and Innovation in Health (CEIH)
Quick Facts:
Location: South Australia (SA)
Agency: Commission on Excellence and Innovation in Health (CEIH)
Health System: SA hospitals handle approximately 2,240 admissions per hospital and 16,150 emergency presentations daily
Workforce: 44,000 registered health professionals on the frontline, excluding operational and functional support staff
Focus: Automating decision-making processes to reduce cognitive load on clinicians through a learning health system
Partners: All universities in SA, metropolitan Adelaide's local health networks, Health Translation SA, SA Department of Health, and Digital Health and Chief Medical Information Officer's office
The Challenge: Reducing gaps in decision-making and clinical variation
CEIH, in partnership with key stakeholders, faced the challenge of reducing unwarranted clinical variation in general medicine across hospitals in South Australia. General medicine is often the domain where complex patients with multiple conditions, such as heart disease, diabetes, and respiratory issues, are treated. Clinicians in this field handle complex cases where patients’ conditions require careful coordination between various treatments and monitoring efforts.
The problem lay in the unwarranted variation in care delivery. Unwarranted variation refers to discrepancies in care that cannot be explained by the patient’s condition or needs. For example, different clinicians might make different treatment decisions based on subjective interpretations, leading to inconsistencies in care quality. This variability can lead to inefficiencies, increased costs, and suboptimal patient outcomes. The project identified the need for streamlined, data-driven support to reduce this variability and optimise clinician decision-making.
The Solution: Implementing Data-Driven Decision Support
CEIH’s project focused on leveraging data analytics and machine learning (ML) to support clinicians in making informed decisions, particularly in the general medicine domain. The overarching goal was to create a “learning health system” where clinicians could rely on real-time, data-driven insights to reduce variation and improve patient outcomes.
The project focused on three key areas:
- Patient Flow into Emergency Departments (EDs): The aim was to enhance the efficiency of patient transitions from ED to wards and other units.
- Admitted Patient Care: The project focused on improving workflow once patients were admitted to the hospital, ensuring timely diagnoses and appropriate care interventions.
- Transitioning Patients Back to the Community: Ensuring safe and efficient transitions from hospital care back into the community setting.
This collaborative effort included CEIH, local health networks, universities, and other health bodies. They aimed to create evidence-based models that clinicians could use to make better decisions and understand where interventions were effective, where they could be scaled up, and where they needed to be stopped or adjusted.
Design Features Based on Clinician Needs
The machine learning tool for frontline decision-making was developed with a focus on meeting clinicians' needs for accurate, real-time, and actionable insights to support patient care. From the outset, the production process was collaborative, involving close consultation with clinicians to ensure that the tool addressed practical challenges they face in fast-paced, high-pressure environments. Clinicians highlighted the need for a system that could provide clear, interpretable data without overwhelming them with complex analytics, and the tool was designed with these requirements in mind.
The machine learning system was integrated into the clinicians' existing workflows to minimise disruption. It was developed to provide real-time predictions and recommendations at critical decision points, using data from electronic health records (EHRs) and other clinical inputs. This seamless integration allowed clinicians to access data-driven insights without the need for extra steps, ensuring that the tool was user-friendly and easy to adopt. Moreover, the interface was simplified to present information in a clear, digestible format, avoiding technical jargon and focusing on patient outcomes.
The developers worked to ensure that the tool was interpretable and transparent, allowing clinicians to understand how the system arrived at its recommendations. This was crucial for building trust and ensuring that the tool was viewed as an aid rather than a replacement for human judgment. Additionally, the machine learning algorithms were designed to be flexible and adaptable to new data, enabling continuous improvement as the system learned from ongoing use. By providing reliable, timely support tailored to individual patient cases, the tool empowered clinicians to make more informed decisions, ultimately improving patient outcomes and reducing cognitive load on healthcare professionals.
Machine Learning Implementation: Challenges and Solutions
The project utilised machine learning (ML) to analyse large amounts of clinical data to identify patterns in care delivery. Specifically, it targeted reducing unwarranted clinical variation in areas like sepsis diagnosis and treatment.
Three Challenges Encountered:
- Data Quality: One of the most significant challenges was ensuring that the data used to train ML models was of high quality. In many cases, healthcare data is incomplete, inconsistent, or not immediately available in a usable format. To address this, the team worked closely with clinical staff to identify key pain points and data gaps.
- Building Trust in ML Models: Clinicians often hesitate to adopt machine learning systems if they don’t trust the data or the insights provided. To overcome this, CICEIH used an iterative process, presenting early versions of the data models to clinicians and refining them based on their feedback. This interactive approach helped build trust in the data and the machine learning outputs.
- Capacity and Resources: With multiple projects and limited resources, ensuring clinicians had the time to engage with the data models and provide feedback was a challenge. However, early adopters of the ML models became champions of the initiative, helping to increase engagement across the system.
Three Strategic Solutions Implemented:
- Iterative Process: The team began with approach 2 (traditional benchmarking) but quickly moved into an iterative process (approach 1) where they sat down with clinicians to understand their specific problems. After collecting feedback, they built a data model and then presented it back to the clinicians for validation. This cycle repeated until a satisfactory model was achieved.
- Targeting Specific Diagnoses: The team focused on specific diagnoses where there was a clear opportunity to reduce variation, starting with sepsis. Sepsis, a potentially life-threatening blood infection, requires timely diagnosis and treatment. By using machine learning, the team was able to predict sepsis more accurately, improving patient outcomes.
- Real-Time Data Integration: One of the breakthroughs in the project was the ability to build models that incorporated real-time data, ensuring that clinicians had access to up-to-date information at the time they needed it most. This improved the relevance and accuracy of the ML models in real-world clinical settings.
Outcomes: Improved Care and Efficiency
The implementation of machine learning models in the SA health system yielded significant positive outcomes:
Reduction in Unwarranted Clinical Variation: By providing clinicians with data-driven insights, the project helped reduce unnecessary variation in patient care. The machine learning models allowed for more consistent decision-making across different hospitals and clinical settings.
Enhanced Sepsis Diagnosis: One of the standout successes was in the early detection of sepsis. The ML models were able to predict sepsis more accurately and at an earlier stage, leading to faster treatment and better patient outcomes. The timely intervention reduced mortality rates and improved recovery times for patients with sepsis.
Increased Efficiency in Patient Flow: Machine learning also contributed to optimising patient flow within hospitals. By streamlining the diagnostic process and reducing unnecessary delays, patients were able to receive appropriate care more quickly, reducing their length of stay in hospitals.
Improved Clinician Engagement: The iterative, feedback-driven approach helped build trust between clinicians and the data science team. Clinicians felt more confident in the machine learning models, leading to better adoption rates and more sustained use of the tools in daily practice.
The Future: What’s Next for CEIH and the Health System?
The project’s success in reducing unwarranted clinical variation has laid the foundation for broader implementation of machine learning across other areas of the SA health system. Moving forward, CEIH plans to expand the scope of machine learning to cover more medical conditions and care pathways.
The following initiatives are on the horizon:
- Further Refinement of ML Models: While the sepsis prediction model is performing well, the team is working to refine it further and apply similar models to other high-priority diagnoses. This includes expanding the use of ML to areas such as respiratory and cardiac conditions.
- Clinical Testing and Validation: The next phase involves more rigorous testing of the machine learning models in real-world clinical settings. CEIH aims to ensure that these models provide new, actionable insights to clinicians rather than merely confirming what they already know.
- Embedding Machine Learning into Everyday Practice: Machine learning is just one part of a broader change management process. CEIH plans to continue working closely with clinicians to ensure that ML tools are seamlessly integrated into their workflows and that they receive the necessary training and support to use them effectively.
- Sustaining Change: One of the core goals is to ensure that the impact of these changes is sustained even after the project ends. To that end, CEIH is focusing on building long-term capability within the health system, ensuring that future clinicians can continue to benefit from data-driven decision-making tools.
- Related Events
- Government Innovation Showcase South Australia
- Communities
- Regions
-
Australia
Published by
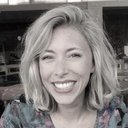
Related
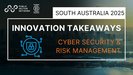
Industry Trends
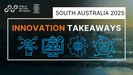
Industry Trends
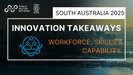
Industry Trends
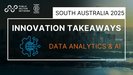
Industry Trends
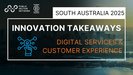
Industry Trends
Recommended
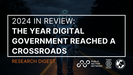
Industry Trends
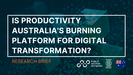
Whitepapers & Reports
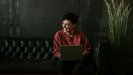
Case Studies
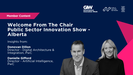
On Demand

Whitepapers & Reports
Sign up
Most Popular Insights
Most Popular Partner Content