AI and the Emerging Trust Challenge
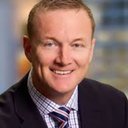
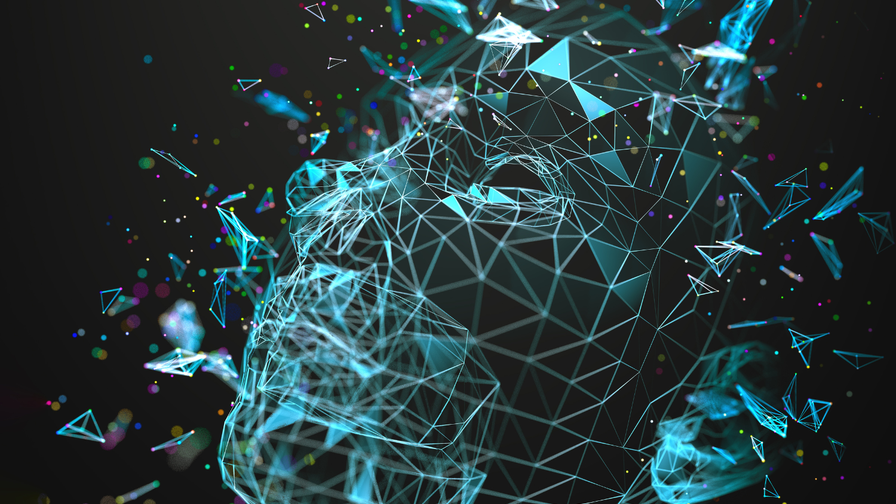
Over the last half a century, digital technology has become indispensable for businesses, governments, and individuals globally. The trust we place in technology is due to rigorous and transparent verification practices that have evolved over many years. Robust methods, tools, standards, and industries have emerged to verify technology, laying the trust foundation by addressing key questions:
• How was the technology designed and how does it function?
• Who built it and how was it built?
• Does it operate as designed?
• How might it fail or cause harm?
• Is the technology vulnerable to exploitation or misuse?
• Is it reliable, scalable, and resilient?
• Is it the genuine item?
• Does the technology comply with relevant standards or regulations?
As we move into an era where #ai is becoming ubiquitously available, new #trust challenges emerge, requiring a re-evaluation of traditional technology verification paradigms.
Provenance: The crux of any AI system is its training data. The creation of AI models is a collective endeavour, either spearheaded by domain experts or driven by cost-efficiency. Transparency and thorough documentation in AI model development are critical.
Interpretability: Understanding AI models is complex. Unlike conventional software, AI operates on probabilities, fuelled by patterns generated from vast datasets. These models generate variable content, leading to unique verification challenges. Despite strides with frameworks like XAI (Explainable AI), a comprehensive toolkit for AI interpretability is yet to be developed.
Authenticity: Ensuring authenticity in the expanding landscape of AI models is paramount. Robust mechanisms are needed to ascertain the authenticity, guarding against subpar replicas or unauthorized alterations. Techniques like Homomorphic Encryption and zkML are promising but early in development.
Adapting to the difference between AI and traditional software demands adaptation of existing processes, methods, and standards. Third-party audits and certification processes are likely to play a pivotal role in nurturing trust in AI systems. Moreover, AI itself will become an indispensable tool for inspecting, testing, and verifying other AI systems. Machine learning models will pinpoint anomalies, biases, or potential failures in other AI systems, aiding in generating testing datasets and automating the continuous monitoring of deployed AI systems.
Trust in AI will be critical for its success and acceptance, akin to traditional software. The journey is young but full of promise and opportunity.
- Communities
- Digital Services and Customer Experience
- Tags
- #ai #trust
- Region
-
Canada
Published by
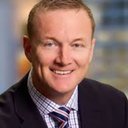
Most Popular