Adaptive Data Architecture For Responsible & Safe AI Adoption in Government: Reflections & Learnings from 2023
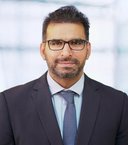
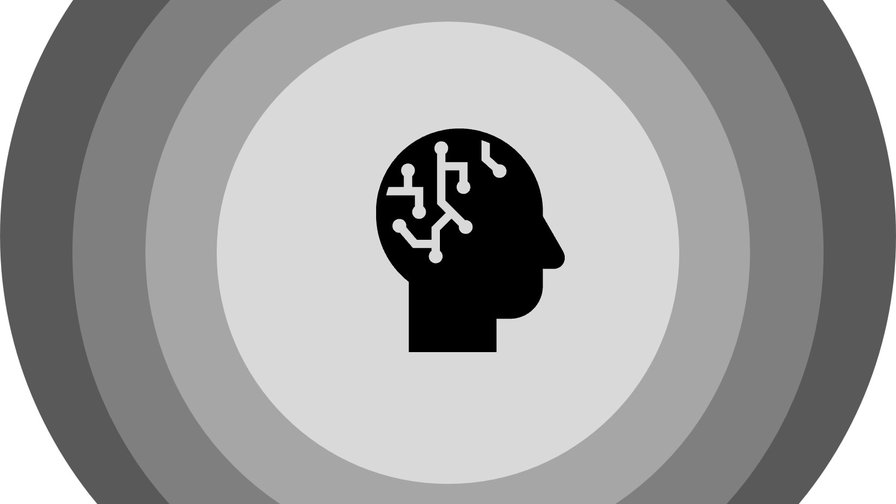
There is an increasing interest, noise and hype around AI (Artificial Intelligence) and GAI (Generative AI) adoption in public sector. I attended several conferences in 2023, reviewed several reports, presented, exchanged and involved in several round tables and debates on this topic (e.g. [1-10]). This article presents key reflections and learnings from 2023 and proposes next steps for establishing the adaptive data architecture for the responsible and safe AI adoption in public sector.
Human vs. AI/GAI system: Principal-agent relationship
Let's talk about what is not an AI/GAI system. Our typical data analytics and reporting are not AI/GAI systems. AI systems exhibit human like capabilities such as anomaly detection, learning, reasoning, speech and vision etc. GAI systems, an extension of AI, automatically generate contents such as text, images, policies, recommendations, reports and can make human like conversations. It is important to clarify that we should not fear and assume that an AI/GAI system is a human, or it will replace the human. AI/GAI systems should not assume or force to replace humans or have human like emotional, physical or spiritual needs.
AI/GAI system is an agent or a digital assistant of a human. Principle delegates the tasks to the agent. Thus, there is a principal-agent relationship (delegation) between the human being the principal and AI/GAI system being the agent who carries tasks or acts on the behalf of the principal (human) based on the agreed contract. Such contract can be built into the AI/GAI system and should be monitored by the human (self-monitoring) or another third party or monitoring system (e.g. AI system governance and assurance).
OECD provides an updated formal definition of an AI system, and defines AI system as:
"a machine-based system that, for explicit or implicit objectives, infers, from the input it receives, how to generate outputs such as predictions, content, recommendations, or decisions that can influence physical or virtual environments. Different AI systems vary in their levels of autonomy and adaptiveness after deployment.” [1]
AI is not new. So what is new, and why now?
AI is not new. The history of AI can be traced back to 1950s [2]. What is new here is the focus on mobility & digitalisation of staff and citizen interactions & services; commoditisation of AI research & models; connectivity & online access; changing society, lifestyle, & habits; and the recent widespread hype of GAI capabilities around content generation and their positive and negative impacts etc.
AI threat and opportunity
The use of AI/GAI in public sector offers several uses cases and associated benefits (e,g, productivity, decision automation, fraud and scam detection). Following are the examples of AI use cases in public sector.
- It can be embedded in the citizen interactions with government agencies (citizen lifecycle or journey).
- It can be used to capture conversations and notes during interactions.
- It can be used to translate contents from one language to multiple languages.
- It can be used in intelligent call routing/service centers and chatbots/conversations to solve issues and tickets.
- It can be used to develop and test software applications.
- It can be used use to detect fraud and scams.
- It can be used for creative writing and visualisation.
While AI/GAI offers several benefits and uses cases, it also has potential harms to citizens and government (e.g. human safety, government trust/ reputation).
- It may be used as a fake identity or voice impersonation.
- It may pose physical or eSafety issues.
- It may use static or outdated datasets.
- It may use copyright data without permission.
- It may not have access control over the inputs and outputs (e..g LLM and its inability to control over their data inputs or outputs)
- It may learn unexpected or unethical behavior.
- It may not unlearn or forget, or unlearning may require re-training the AI models from the scratch, which may incur additional cost.
Citizens and government's roles in AI adoption
It is not the responsibility of the government only to ensure the responsible and safe adoption of AI. Both the citizens and government need to play their roles for the responsible and safe AI-enabled Society.
AI system can be used as a personal digital assistant by citizens. They should use it responsibly and ethically for legitimate tasks for fair gain though fair means. Citizens should not interact with the AI systems in a way to train the AI system or make it learn unethical behaviour. Citizens report any unethical or unlawful behaviour (self-governance) to relevant AI government authorities.
Government can play different roles. As a regulator, they need to provide necessary AI laws and ensure their enforcement via AI law enforcement bodies. As a facilitator, they need to provide AI infrastructure, guidelines, polices, principles, frameworks and drive the right signals for responsible and safe AI adoption [3-5]. As a consumer, they need to prioritise responsible and safe use of AI in government service offering and interactions with citizens (also staff), while considering the diversity and equality.
AI adoption and the data challenge
What is stopping or impeding the AI adoption in public sector? There are several social and technical challenges including the fear of unknown consequences. However, one of the repeatedly observed challenges is the 'right data foundation,' which is critical for the adoption of AI. Following are the key data challenges.
- Difficulties with right data acquisition (real-time, non-real time), believability, consistency and quality.
- Difficulty with data governance, observability and provenance.
- Toxic data, which is not useable or dark data, which has not been used.
- Siloed and duplicate data
Adaptive data architecture as the solution
A strong data foundation is needed to address AI adoption related data challenges. There is need to establish flexible data architecture, which is adaptable to the evolving AI system needs and data landscape. Adaptive data architecture can make use of modern enterprise knowledge graph (EKG)/semantic virtual layer (SVL) for connecting curated federated data sources rather having a bulky traditional centralised data warehouse or toxic and dark data holdings in the dirty data lakes.
Data governance and quality assurance can be implemented at once at the data source level (federated data governance and quality). AI systems can connect to the semantic virtual layer of the data architecture and discover, and use, the well governed and quality assured data for trusted prerational and non-operational intelligence.
Conclusion and next steps
What is next? Look at AI adoption from a whole of a government ecosystem perspective where government is made of different layers, underpinning services and supporting agencies (e.g. federal, state, local). However, there is a need for a unified front and platform for citizens to interact with digital government (non-AI and AI enabled) services. Here are the key take outs.
- Design adaptive government data ecosystem architecture to facilitate the implementation of AI proof-of-concepts (PoCs) and trials using the secure AI system development guidelines [7] and related processes [8] for the selected consequential (e.g. high value or high risk) use cases [6] for the selected service areas, associated government agencies and sectors (e.g. customer service, transport, health, education).
- Establish and manage AI use case inventory across the government ecosystem including data catalogue and connected AI application portfolio.
- Evaluate the AI PoCs results and collect evidence to update the existing regulations and polices in each government sector, and only define new regulations and polices if needed (must have). Too many regulations and polices may hinder the adoption of AI due to the possible confusion, unnecessary complexity, overlap and their enforcement, monitoring and governance cost. Settling on generic regulations early (due to the fear of AI) without evidence (e.g. not having any AI PoCs/trials/ experiments) may not be practical and feasible.
- Establish AI good governance and guidance boards and supporting committees such as AI Audit, AI Risk, AI Ethics, AI Innovation, AI Technology and AI Investment committees while balancing AI governance, risk, compliance and innovation.
- Develop and make use of relevant frameworks such as AI Assurance [4] and Highly Consequential AI Use Case Selection [6] frameworks.
- Establish AI taskforces to interface with different agencies, industry and academia for developing and sharing guidelines, best practices, and patterns of AI adoptions. This is important for collective action/responsibility and learning about new AI technology.
- Facilitate social governance via the establishment of an independent AI advisory committee, engaging communities of practice, and academia to ensure accountability and trust.
Key References & Resources:
- OECD (2023). Updates to the OECD’s definition of an AI system explained.
https://oecd.ai/en/wonk/ai-system-definition-update. - Turing, A. M. (1950) Computing Machinery and Intelligence. Mind 49: 433-460.
- Supporting responsible AI: discussion paper.
https://consult.industry.gov.au/supporting-responsible-ai - Artificial intelligence assurance framework.
https://www.digital.nsw.gov.au/sites/default/files/2022-09/nsw-government-assurance-framework.pdf - Australia’s AI Ethics Principles.
https://www.industry.gov.au/publications/australias-artificial-intelligence-ethics-framework/australias-ai-ethics-principles - Framework for Identifying Highly Consequential AI Use Cases
Framework for Identifying Highly Consequential AI Use Cases - SCSP. - Secure AI system development guidelines.
https://www.ncsc.gov.uk/collection/guidelines-secure-ai-system-development - FTC Authorizes Compulsory Process for AI-related Products and Services
https://www.ftc.gov/news-events/news/press-releases/2023/11/ftc-authorizes-compulsory-process-ai-related-products-services - Adoption of Artificial Intelligence in the Public Sector.
https://architecture.digital.gov.au/adoption-artificial-intelligence-public-sector-0 - Artificial Intelligence in the Public Sector.
https://documents1.worldbank.org/curated/en/746721616045333426/pdf/Artificial-Intelligence-in-the-Public-Sector-Summary-Note.pdf
- Communities
- Digital Services and Customer Experience
- Region
-
Australia
Published by
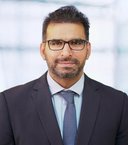
Most Popular