Accelerating Citizen Outcomes by Transforming Data into Value with Data Science
Government Keynote:
Leveraging machine learning to glean detailed insights from qualitative as well as quantitative data
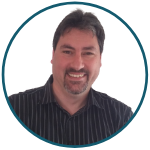
Stefan Kuegler
Associate Director,
Data Science & Visualisation
NSW Department of Premier and Cabinet
Developing a data science mind-set and team
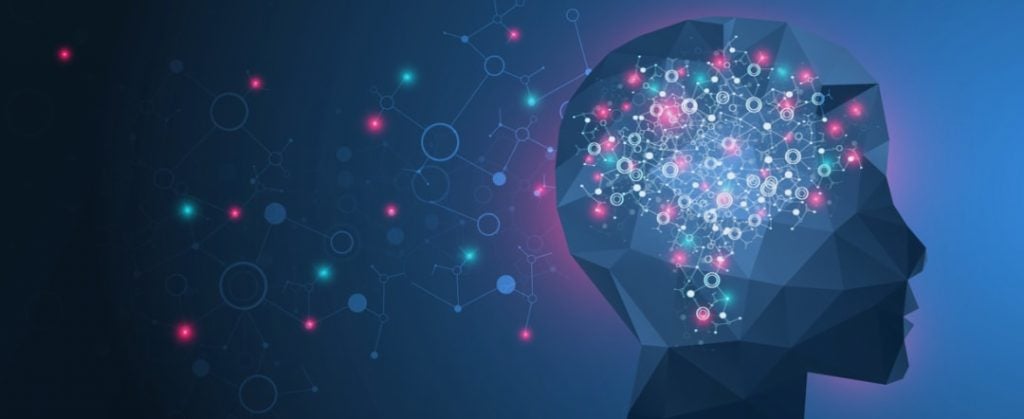
Most organisations, especially ones that have or produce large amounts of data, are constantly looking to ease the analysis process, and take some of the manual and repetitive steps out of it. In the past, this was difficult to do because the technology wasn’t up to it, but now, as technology continues to develop and evolve, there are greater options for automation.
Stefan Kuegler
, the
Associate Director of Data Science and Visualisation
at the
NSW Department of Premier and Cabinet (DPC),
says that recently, they have been able to “leverage machine learning to glean detailed insights into qualitative as well as quantitative data.” This has been a long and complex process but has produced positive results.
“The DPC had long had a data team, but “we were not really a data science place. We were very much just an analytical team.” A department like DPC has a constant flow of data coming in, and analysing it efficiently and appropriately is the role of the data team. They were good at their job, “but we found that there was more that we could be doing with the data to really exploit that data in a better way. We also needed to do it quicker.” There was a lot of talk around the team about how this could be achieved, and the creation of a data strategy was proposed, “though what we actually needed was an overarching data science strategy.” It became clear that “data science was evolving and really taking off.” They were a “little unit” doing their own thing, but decided that is where they needed to be.”
Out of their little team, they decided to build a data science unit. This started with the establishment of appropriate “reporting lines and a structure.” That allowed them “to take other people along on the journey as well,” and ensured they had buy-in from the senior executive. Once the foundations were laid, it was all about “showcasing little wins along the way.” For data science to really be effective, it has to work with live, current data, and has to prove that it can be efficient. Analysing data from months ago, or projects in the pipeline are great, but especially when setting up, something current shows all involved that what is being initiated is worthwhile. Therefore “education was a huge part of this – not only of our own people, but also education for the people who are interested in what we do as well.”
Since the original team was small and quite niche, “our recruitment strategy also changed from hiring just analysts, to hiring data science specialists or experts. It was really important for us to put the right people in the right place at the right time.” Then once the foundations were set and the experts were in place, “ we allowed the people an ability to play. We gave them the data that we needed and gave them the chance to service it in their own time.” At least initially, it wasn’t about timeframes or “an agenda for individual projects. We hired people for their skills, and we wanted to see what they could do.”
Data challenges and misconceptions
Although there was buy-in from the whole department, that doesn’t mean that they were constantly supportive. Before education around data science commenced, many people – including colleagues from other teams – “questioned what we were doing.” Some people accused them in a derogatory way of “just playing with data.” It is true that they were ‘playing’ with it, but for a very specific purpose.
The other misconception was that once machine learning was applied, everything would change and speed up instantly. Even the experts within the team needed to do “a lot of learning, education and expertise training.”
Moreover, there was a perception that machine learning was about setting and forgetting, and that it would do everything, almost without even the need for human involvement. “But a lot of the time we still need to be sitting there in the background and helping guide it to get the information we are after, something that’s usable.” The machines can perform a lot of tasks quickly, but they still need to be taught what to do and what results are being sought.
Benefits of machine learning
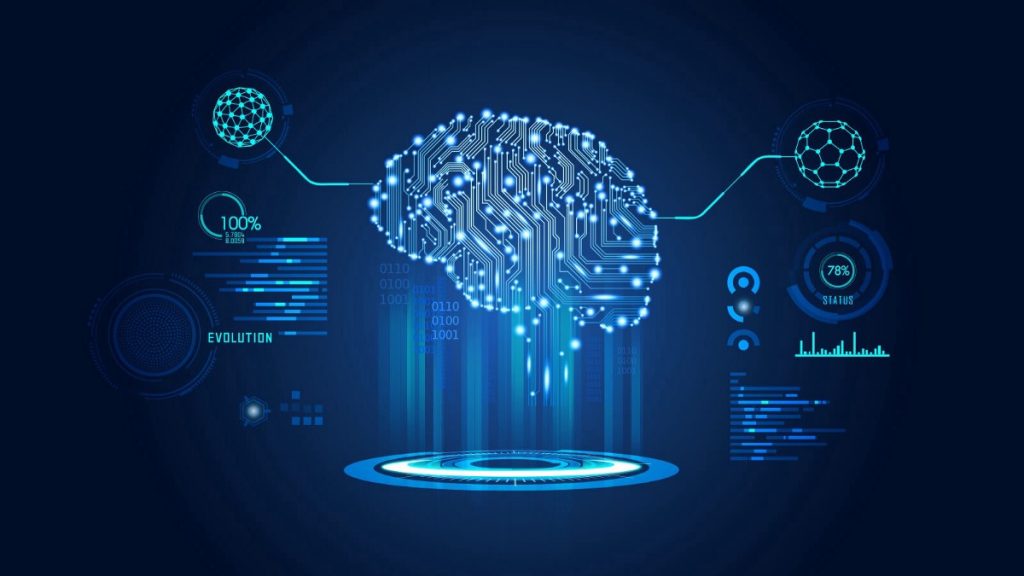
Though there were barriers initially, eventually the machines were set up and ready to produce results. The machines were set up in the first place because DPC collects a lot of data, particularly qualitative data with a significant amount of free text. Before the machines, “much of the qualitative data was turned into quantitative data for better analysis,” but generally that meant that things like “subjectivity, context, and the interpretation of what people were really saying was lost.”
"
We looked at machine learning to see if we can speed up some of the processes that we do in order to get richer and deeper insights from the data, and also to use more of the data. It was about combining the machine with our innate human ability to understand, and using the machine learning to speed up some of our processes, especially the repetitive ones.
Stefan Kuegler
Associate Director, Data Science & Visualisation, NSW Dept. of Premier and Cabinet
Humans were always intended to be part of the process. It was never about replacing humans with machines, but it was about “accentuating and accelerating our ability to get information out. And it was also about replicating and repeating the processes.” Some of the perceptions was that it would do everything, “but we had to remind people, that as the name suggests, it is still learning.”
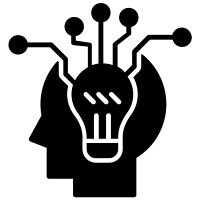
For the DPC, machine learning was generally for text analysis in three specific areas:
- Sentiment analysis – “Looking at that positive or negative context of what people say and trying to understand how that fits across the overarching feedback that people are giving us.”
- N-grams – “Looking at key phrases and seeing where those phases are used the most.”
- LDA (Latent Dirichlet Allocation) – “Looking at words and clustering them together.”
Each of these processes required algorithms and time to set them up, and would have taken “many weeks” to analyse manually. The machines gave results speedily. Some data is still being processed in new ways so not all the results are in, but the sentiment analysis shows “not only the overarching sentiment, which we can build up, but we can also look at individuals and see how they compare.”
In terms of the LDA analysis, “we’re looking at those clusters and trying to see what are the main themes that are coming out. These clustering methods allow us to go very quickly into what is the theme that people are trying to convey.” It allows the analysts to look at things holistically or to drill down as far as they want to, based on the algorithms that they build. In terms of sentiment analysis, since that is related to particular words or phrases, it is “built around various dictionaries,” but some of them are quite limiting and specific to a region, “so we might be building our own.” Each tweak takes time to develop and produces different results, so having expertise is critical.
Having the right people in place has been crucial because part of the analysis has been about understanding the background to the data in the first place, and then creating the machine processes for that.
This process of machine learning for text analysis is not only much faster, “but it allows us really to go back into the data and to provide us a better understanding of the information, with better insights.” It allows real stories to emerge and ensures that no data is wasted. This process has been successful because “we got the right people for the right data,” with ample time to set it up and play with it. “We were also lucky that we had a ready-made topic so that we could start with data straight away.” Anyone who doubted the team soon saw results, and soon saw the benefits. In fact, it has been so successful that “in the future it will probably allow us to look at different unstructured data, such as social media and even reviews.” Using data for “real analytics and for good” has always been the goal.